Brain-Computer and Brain-Machine Interfaces (BCI/BMI)
BCI/BMI enable direct communication between the brain and external devices. These systems translate brain signals into commands to control computers, prosthetics, or robotic devices, offering potential in rehabilitation, cognitive enhancement, assistive technologies and even in entertainment or gaming applications.
Setup Requirements and Technological Challenges
The combination of accessibility, safety, and real-time monitoring makes EEG an ideal option for many BCI applications. EEG systems can provide a real-time brain data stream to control external devices, while being non-invasive, affordable, and relatively easy to set up compared to other brain-monitoring methods like invasive implants or functional MRI. A typical EEG-driven BCI/BMI system consists of the following components:
EEG Sensors
An array of electrodes placed on the scalp to detect electrical signals generated by neural activity. A higher concentration of electrodes near the cortical area engaged by the task can be beneficial to detect fine-grained activation patterns.
Signal Amplification
High-quality electronics capable of amplifying the brain's electrical signal while maintaining its integrity and minimizing noise and artifacts. This module should be portable and possible to integrate into the output device (e.g. exoskeletons or power wheelchair), in order to be suitable for practical, everyday applications.
Signal Processing Unit
A dedicated software to implement online processing of the data (e.g. filtering, artifact removal, feature extraction) is a key component in BCI. While this is essential to isolate meaningful brain activity relevant to the task, it has to be performed in real-time to prevent latency issues and the introduction of noticeable lag in the command being controlled.
Machine Learning Algorithms
Decoding brain activity into actionable outputs is a crucial step. Machine learning algorithms are employed to classify different brain states or intentions based on the EEG data. These algorithms must however be trained by the user on vast datasets to improve accuracy over time. Furthermore, inter-subject variability limits the possibility of a generalized approach. Since each individual’s brain signals are unique, user-specific training data is often necessary.
Output Device
Once processed, the decoded signals are sent to the output device, whether it’s a computer cursor, robotic arm, or another machine that executes the user's intended actions. One of the main challenges is the long-term stability of the hardware, which should perform consistently without requiring cumbersome or frequent recalibration.
Our Solutions
Our eego™ and waveguard™ portfolios offer highly customizable solutions for BCI applications, ranging from ultra-portable 8 or 24 channel to research-grade 64 channel amplifiers, in combination with different sensor types (gel, sponges or dry) to suit the most diverse set-ups. Our SDK allows you to import the EEG data stream directly into your C, Python or Matlab-based script. ANT Neuro systems are also compatible with BCI platforms like BCI2000 and OpenVibe.
Showcases and Publications
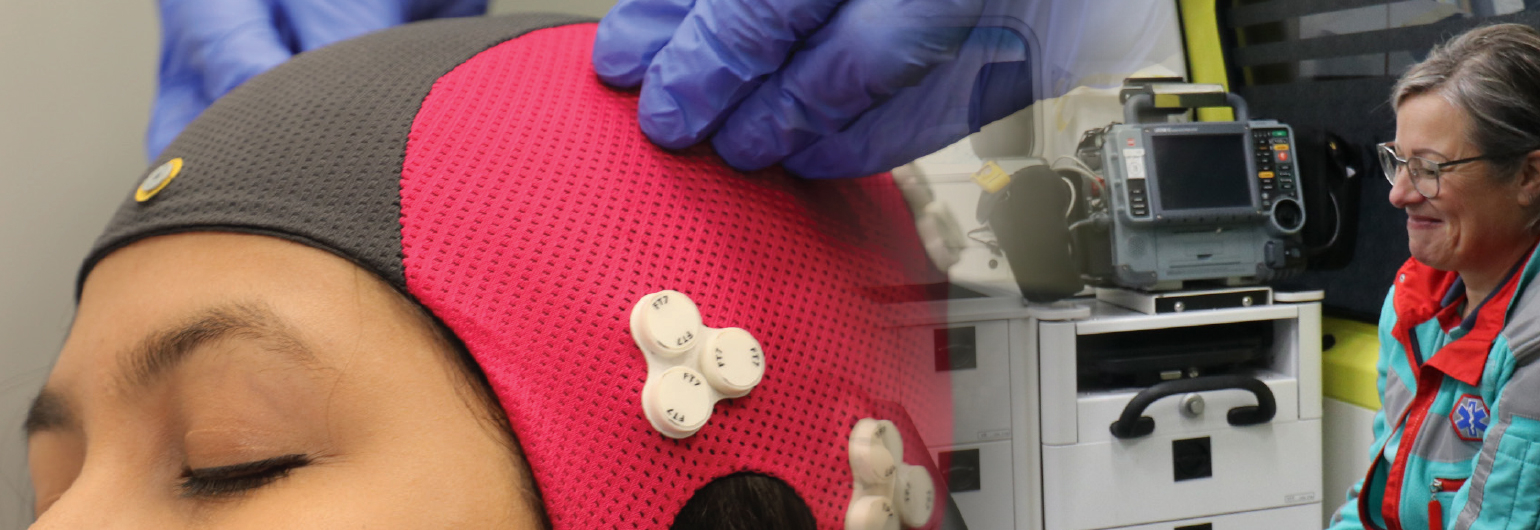
The MindWalker Project
EEG and EMG in rehabilitation. In this application case we elaborate on the application of the eego sports system in rehabilitation settings.
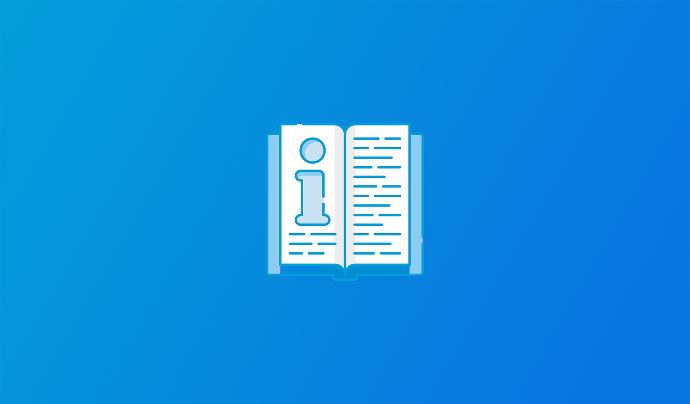
A computational framework to investigate the effect of robotic assistance on human muscular effort
Emel Demircan, Sorumlu Yazar
Read More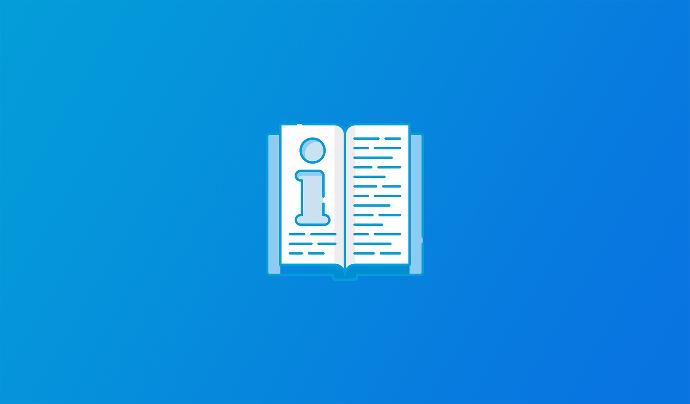
ROS-Neuro: A common middleware for BMI and robotics. The acquisition and recorder packages
Tonin, L., et al.
Read More