Neuromarkers and AI
Modern neurotechnology research demands state-of-the-art technology to efficiently deploy machine learning algorithms, such that the benefits of AI can be leveraged for social good and betterment of quality of life. Use-cases that target predictive prognosis for dementia, classifying the EEG signal for precise triaging in case of urgent hospitalization needs, home-based self-diagnostics or managing cognitive decline in older adults for their well-being can all benefit from the recent advancements in AI.
Setup Requirements and Technical Challenges
Most applications that make use of AI approaches include physiological data (such as EEG, EMG etc) which is recorded with an EEG amplifier and headset, with a labelling or classifying process that is done prior to feeding the data as an input to the model. AI is often used to support human decision-making, primarily in healthcare and medicine, and the black box approach has growing concerns as it is important to learn how decisions were formed in the first place.
Optimization to training data can lead to unintended consequences
There have been cases of wrong AI predictions in cases of skin lesion malignancy as the markers on the images used by dermatologists led to wrong classification. Any kind of markers on physiological data therefore needs to be precise and free of operator errors as much as possible.
Quality of input datasets
All AIs are trained on large datasets and the data collection and labelling should be done carefully to avoid a dataset of low quality being used to train the classifier. The EEG equipment used for data collection is therefore a prime factor.
Bias in the existing data set
Popularly used datasets are often heavily biased towards data from US and Western Europe while other populations are not well-represented.
No viable non-invasive biomarkers for most prediction algorithms
This remains a challenge for most studies that investigate early onset of a disease or disorder.
Our Product Recommendations
While the challenges related to the algorithm or classifiers can only be resolved with advancements in ability to apply a method (unsupervised vs. supervised learning, deep learning etc) that gives us the best outcomes, to improve the data quality and collection procedure is well within our control. The eego™rt range of solutions allow users to create their own custom platform using our software development kit (SDK). With this, users can tailor the recording and labelling phases to their needs. Finally, the SDK files are also provided to all eego™ solution users to allow them to explore possibilities beyond those offered by the eego software. Finally, since the eego software is LSL compatible, users have the choice to also stream out the EEG (and additional sensor data) to a third-party platform of their choice.
Showcases and Publications
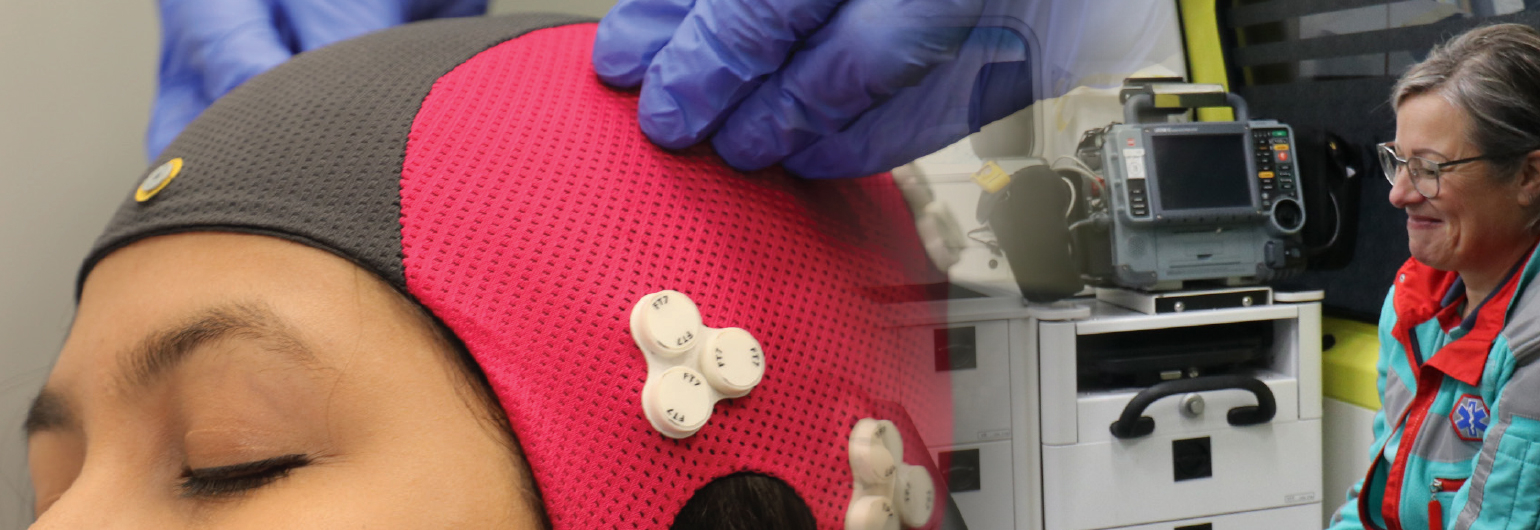
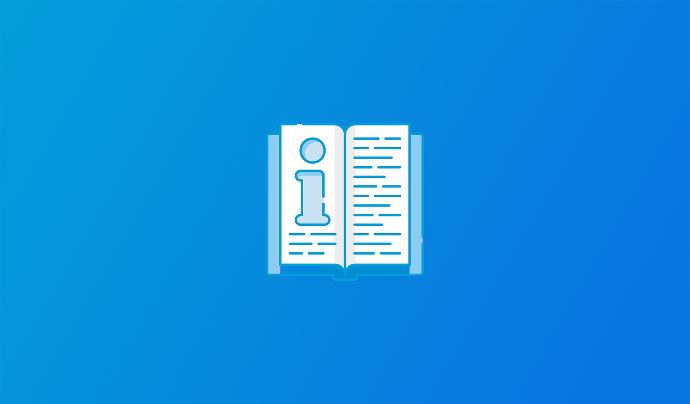
Prehospital Detection of Large Vessel Occlusion Stroke With Electroencephalography: Results of the ELECTRA-STROKE Study
van Stigt, Maritta N., et al.
Read More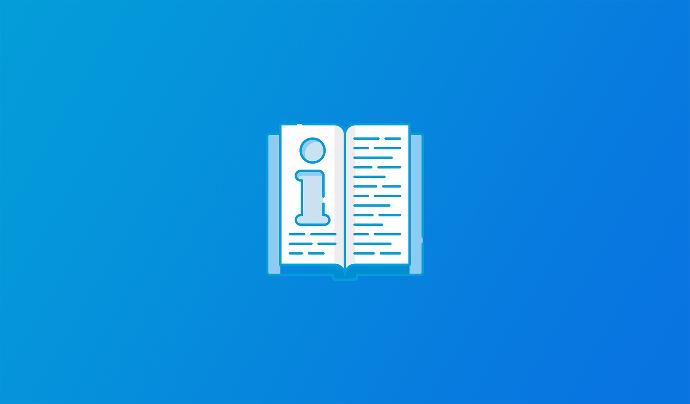
Intelligent digital tools for screening of brain connectivity and dementia risk estimation in people affected by mild cognitive impairment: the AI-Mind clinical study protocol
Haraldsen, Ira H., et al.
Read More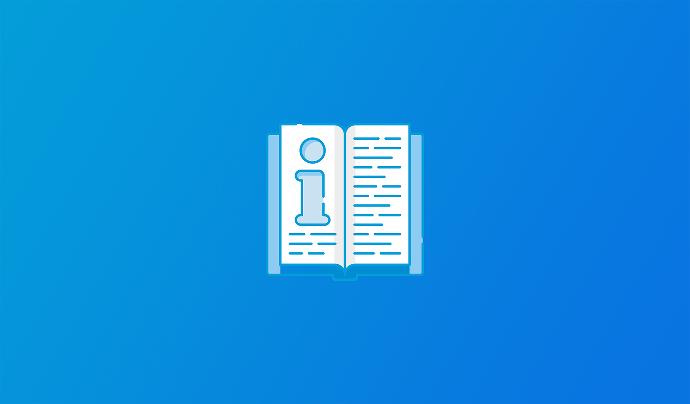
Deep Learning Approaches for Electroencephalography (EEG)-Based User Response Prediction
Sharma, Greeshma, et al.
Read More